Revolutionizing Healthcare with Medical Image Annotation
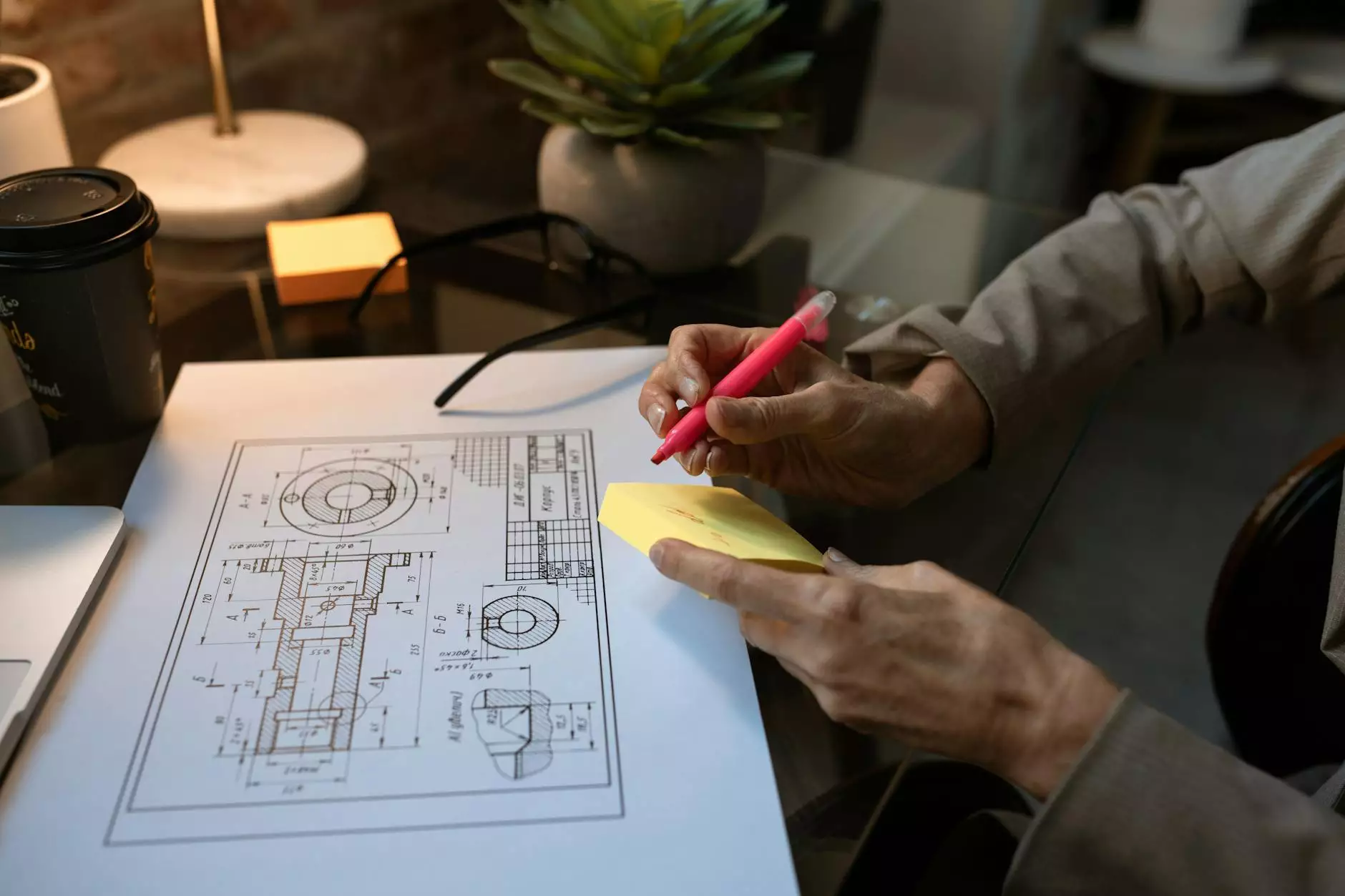
The healthcare industry is constantly evolving, fueled by advancements in technology and the increasing demand for enhanced patient care. Among these innovations, medical image annotation has emerged as a vital tool that is transforming diagnostic imaging and patient treatment protocols. In this article, we will delve into the intricacies of medical image annotation, its significance, and how it can benefit both healthcare professionals and patients alike.
What is Medical Image Annotation?
Medical image annotation involves the process of labeling and tagging the different parts of medical images, such as X-rays, MRIs, and CT scans. This process enables computers and healthcare professionals to identify and interpret various anatomical structures, lesions, and abnormalities in these images with higher accuracy. By utilizing machine learning and artificial intelligence technologies, medical image annotation simplifies data management and analysis, leading to improved diagnostic processes.
The Importance of Medical Image Annotation in Healthcare
Medical image annotation serves several purposes, greatly influencing healthcare delivery and patient care. Here are some key advantages:
- Enhanced Diagnostic Accuracy: By annotating medical images, healthcare professionals can better identify pathological conditions, leading to quicker and more accurate diagnoses.
- Improved Treatment Planning: With precise image annotations, doctors can tailor treatment plans based on specific conditions identified in the imaging studies.
- Training and Education: Annotated datasets are pivotal for training medical professionals and developing algorithms in machine learning, enhancing their skills and the overall quality of care.
- Research and Development: Medical research benefits from annotated images, allowing for large-scale studies and the advancement of clinical understanding through data analysis.
How Does Medical Image Annotation Work?
The workflow of medical image annotation can be broken down into several critical stages:
1. Image Acquisition
The first step involves capturing medical images using various imaging modalities. These images are the foundation for subsequent analysis and annotation.
2. Image Preprocessing
Before annotation, images may need to be preprocessed to enhance quality. This can include adjusting contrast, noise reduction, and normalizing image sizes to ensure consistency.
3. Annotation Process
During this stage, trained annotators use specialized software to label key features within the images. This may involve:
- Bounding Box Annotations: Often used for identifying and outlining tumors or lesions.
- Segmentation: This involves delineating the exact boundaries of organs or abnormalities.
- Landmark Annotation: Used for identifying specific anatomical points of interest.
4. Quality Assurance
Once annotated, images undergo a quality assurance process to validate accuracy. This step is critical to ensure that the labels reflect true anatomical and clinical information.
5. Integration with AI and ML
Annotated datasets are often used to train artificial intelligence and machine learning models, enabling these systems to recognize patterns and improve diagnostic capabilities autonomously.
Applications of Medical Image Annotation
The applications of medical image annotation are broad and impactful. Here are some of the notable areas where it is making significant contributions:
1. Radiology
In radiology, annotated images are crucial for detecting various ailments including tumors, fractures, and other pathological conditions. Accurate image interpretation leads to better treatment options and outcomes.
2. Oncology
In oncology, medical image annotation helps oncologists plan treatments by providing critical data about tumor size, location, and response to therapy over time.
3. Neuroscience
Neuroscientists utilize this technology to study brain structures and identify changes related to neurological disorders, enhancing research into diseases like Alzheimer's and multiple sclerosis.
4. Orthopedics
In orthopedics, annotated images assist in diagnosing joint diseases, fractures, and other musculoskeletal issues, improving patient care through proactive management strategies.
The Role of Technology in Medical Image Annotation
With the evolution of technology, medical image annotation has become more efficient and accessible. Innovations such as:
- Cloud Computing: Allows for storage and sharing of large datasets across platforms, enhancing collaboration.
- Deep Learning: Automates parts of the annotation process by training systems to recognize patterns and make annotations.
- User-Friendly Software: Enhanced interfaces and tools expedite the annotation process for professionals, allowing them to focus on critical analysis rather than cumbersome tasks.
Key Considerations for Effective Medical Image Annotation
For medical image annotation to be most effective, several factors must be considered:
1. Accuracy and Consistency
Ensuring that annotations are accurate and consistently applied across different datasets is paramount. High-quality training of annotators is essential to achieving this goal.
2. Continuous Updates
As medical knowledge evolves, so too should the annotations. Continuous updates are necessary to reflect the latest medical standards and findings.
3. Ethical Considerations
Patient consent and data privacy are crucial in medical image annotation. Ethical frameworks should guide the use and sharing of sensitive medical information.
Future Prospects of Medical Image Annotation
The future of medical image annotation holds immense potential. As technologies advance, we can expect:
- Increased Automation: Further integrations of AI to automate more tedious aspects of annotation.
- Wider Adoption: More healthcare institutions will recognize the value of annotated images and integrate these processes into their workflows.
- Global Collaboration: Enhanced sharing of annotated datasets can lead to collaborative research efforts and improved global health outcomes.
Conclusion
In conclusion, medical image annotation is not just a process—it's a cornerstone of modern medical diagnostics and treatment planning. Its role in enhancing diagnostic accuracy, improving patient care, and facilitating medical research cannot be overstated. As the industry continues to embrace technological advancements and best practices, the potential for more effective healthcare solutions expands exponentially. For those in the field of healthcare, integrating medical image annotation into practices promises a brighter future for patient outcomes and the advancement of medical science.